
From Covid-19 to Fake News, recent conflicts have clarified the threat of disinformation. Is Artificial Intelligence the answer?
In a recent survey of tech policy experts, 90% agreed that the regulation of online disinformation should be a global priority. It’s easy to see why. In an era where disinformation campaigns have catalyzed insurrections, exacerbated pandemics, and led to lasting economic destruction and reputational damage, we need smart, sophisticated, and powerful solutions.
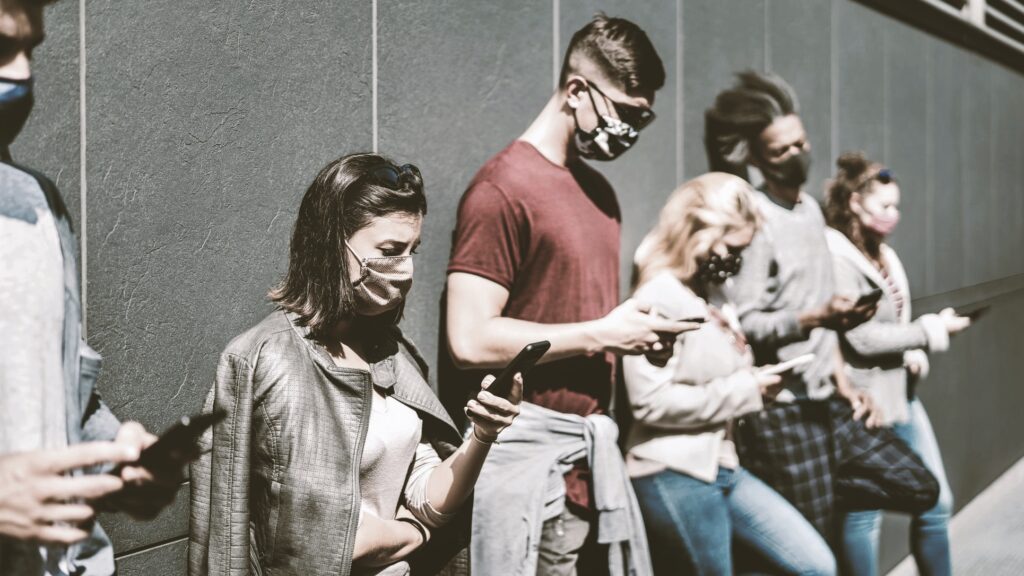
NGOs, governments, militaries, and social media platforms already use AI and machine learning to attempt to detect and counter disinformation campaigns. But AI-supported technologies raise numerous challenges. Tackling those challenges requires a range of expertise, ranging from computer and data scientists to cybersecurity and policy experts, and even lawyers and philosophers.
Let’s look at some of the ethical challenges and risks of harm posed by AI, then consider a few best practices for organizations that need to deploy these technologies.
A Problem of Intention
AI is reliable only insofar it provides a proper filter. We need the filter to avoid both false positives—where data is mistakenly classified as disinformation—and false negatives—where disinformation is allowed to pass through.
To create a reliable filter, the first challenge is determining what counts as disinformation. It should be distinguished from misinformation, which is “honest mistakes”—cases where someone might spread an untruth by accident. By contrast, “disinformation” typically refers to a deliberate intent to mislead others.
This is why organizations concerned with disinformation use what philosophers often call intention accounts. For example, the USAID, a leading international development agency, states that disinformation is “information that is shared with the intent to mislead people.” The European Commission defines disinformation as “verifiably false or misleading information created, presented and disseminated for economic gain or to intentionally deceive the public.” In the U.S., the Department of Homeland Security has defined disinformation as “manufactured information that is deliberately created or disseminated with intent to cause harm.”
All of these definitions seem intuitively on the right track, but things are not so simple.
From a conceptual point of view, intention accounts face serious challenges. What about humor or satire, where people are misled for the sake of a joke? More problematically, intentions to mislead are not always at the heart of acts of disinformation. During the Covid-19 pandemic, for instance, people have spread misleading claims about the disease without having malicious intent. Even so, wouldn’t we want an AI tool to filter life-threatening disinformation?
So, basing our conception of disinformation on intent is not as helpful as it seems. We need more rigorous analysis of what counts as disinformation.
Where AI Can Help—and Where it Can’t
Imagine we have a settled on a definition and want to apply it to filter for disinformation aided by AI. Now we begin to face practical problems. AI can help with the easiest and most repetitive work humans do online. For example, AI can detect and remove dubious content, screen for fake-bot accounts, and find patterns of words that can lead to the identification of false stories (based on what has been flagged by some users as inaccurate). This can help to mitigate the spread of the “easy cases” of disinformation.
AI can even help evaluate intent at some level, thanks to natural language processing (NLP) tools that classify written or spoken data according to writing style, syntactic features, and sentiment.
Though these approaches are promising, they are not fully reliable given the complex nature of disinformation in general. Even checking for the veracity of seemingly misleading statements can be complicated in practice. A piece of data might concern highly judgment-sensitive topics, where there is significant expert disagreement, where the truth and facts are vague, or where there might be information that is still dynamically evolving.
Also, it is tricky for NLP to classify subtle implicatures or claims embedded in complex sentences that rely on cultural nuances and cues. The risks here are serious. Information could be classified as disinformation, and disinformation could fall through the filter.
But to my mind, another significant and underappreciated threat concerns what we might call interface risks. These lie in the navigation of the trade-offs between human judgment and AI technology.
For instance, imagine an analyst going through a large pool of posts on Covid-19 vaccines that have been flagged as disinformation by AI. How much should the analyst rely on their own critical independent judgment? How much should they trust the automated aid and similarity score? How is this line to be drawn?
How is the analyst supposed to deal with difficult cases? When do they refer back to the AI and when do they escalate to another human? When and why are identifications of a disinformation case then used to further train the AI? How are controversial borderline cases dealt with?
Any organization using AI and machine learning technologies to combat disinformation must carefully train analysts to navigate these choppy waters.
Our task is not simply to improve AI, nor to better train humans. Our task is to consistently improve the relationship and interaction between the two.
That’s tough work. But it’s work we can do if we break it down into constituent parts. When an organization comes asking for help with these issues, here’s the path I’d lead them down:
- Define a robust and sound conceptualization of disinformation for the organization’s particular context;
- Clarify where AI can assist and where human judgment remains necessary, with clear lines of authority and responsibility for the interface of interaction between a human analyst and AI;
- Develop sound training for human analysts on disinformation analysis and on the limitations of AI and machine learning;
- Establish a monitoring system for the disinformation detection chain, with an emphasis on improving training sets for machine learning data sets based on a critical review of resolved cases and in light of new data.
AI and machine learning is not (yet) a holy grail for disinformation detection. But we can design for these systems and their successful interplay with the humans who run them and rely on them.